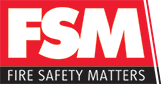
![]() |
Brian Sims
Editor |
Looking at the future
02 May 2019
The Internet of Things (IoT) continues to evolve, transforming the way that video technology is used for surveillance, and cutting-edge hardware now allows video content analysis to deliver more detailed insights. Neil Killick, explains how three key trends are shaping the security industry right now.
HARDWARE ACCELERATION, video content analysis and the IoT are already changing our futures and creating new possibilities. The challenge for all of us is to keep up with the pace of change and understand how they can work together and influence each other. As with any significant technological advancements, there will be winners and losers and the former are leading the way by providing clear insight to customers about how to use this change to their advantage.
Rapid developments in artificial intelligence (AI) mean that soon, the efficiency of machine intelligence will combine with the quality of human judgements to achieve an outcome that’s not possible for either one alone. Machines will carry out most of the work we currently do, with humans assisting when a machine is uncertain.
AI has also initiated a transformation within video content analysis, which will change how video technology is used for surveillance. Traditionally, video analytics has been rule-based with a human programmer setting fixed parameters for every situation that the system must recognise. Next generation video content analysis systems, however, identify everything in a scene, learn about new items as they appear, understand what’s normal behaviour and alert humans to what’s not normal. The efficiency of AI will combine with the quality of human judgements to achieve an outcome that’s not possible for either one alone.
Three technology trends, Aggregation, Automation, Augmentation, are driving this disruption and defining the short-term future for video content analysis.
Aggregation
Sensor aggregation is about joining data from many types of devices to create a whole dataset – it is driven by the IoT and the way it is able to connect vast numbers of cameras and other sensors.
In less than five years, about 50 per cent of the streams feeding into video management systems will not come from cameras by themselves, but will be video streams from other types of sensors. Video content analysis will be about using and analysing this massive input of data and making it actionable. That’s why future video content analysis technology is developing at the central server, because it is not possible to effectively aggregate data at the edge.
This technology is already being used in assembly lines, where a video sensor counts how many components are left in a bin, how fast they’re being used, and then automatically places a supply order to refill it.
The video sensor also helps management to improve the process by providing a constant flow of data to optimise workflows and staffing, revolutionising materials management on assembly lines and creating big commercial gains. Similarly, cities are already using this kind of technology to detect full rubbish bins.
Automation
System automation combines machine intelligence with human intelligence and is being driven by the massively parallel compute capacity that is now available through state-of-the-art graphics processing units (GPUs). Before GPUs, it was impossible to comprehend the information hidden in the massive amounts of data from sensor aggregation. However, GPSs facilitate AI to analyse this data and understand what it is telling us.
Product development is happening at an incredible pace. The Tesla P4 Inference Accelerator from Nvidia is cooled, powered and built to run in a 24/7 high-heat server environment. By combining sensor aggregation with the compute power of GPU technology like the Tesla P4, it is possible to use neural networks efficiently to understand what these huge amounts of video data can tell us.
Neural networks are computer systems used in machine learning and AI. Like a human brain, they are based on a large collection of connected simple units called artificial neurons. Neural networks are not rule-based like traditional systems and, rather than being explicitly programmed, are self-learning processes that can be trained by the user.
They learn what is normal behaviour for people, vehicles, and the environment by observing patterns of characteristics such as size, speed, colour, grouping, vertical or horizontal orientation. By classifying this data, tagging objects and patterns in the video, and continuously building up and refining definitions of what is normal or average behaviour, a neural network can recognise when something breaks the pattern and send an alert.
This technology excels in areas that are difficult to solve using rule-based programming. For example, a neural network learns that container ships sail in approved shipping lanes. When it sees a ship sailing outside of shipping lanes, it recognises this as not normal. GPU and neural network technology are transforming video content analysis and it will soon be possible to reliably find suspects in a crowd, assess situational behaviour and estimate intentions using this method.
Augmentation
By the end of 2014, IHS Data estimated that there were over 245 million operational surveillance cameras globally. In fact, that’s just a fraction of the total number of devices, such as smartphones, that are capturing video. The problem is that most of the video data is rarely looked at because finding exactly what you’re looking for can take a lot of time. The question is how do you search all those hours of video for patterns of behaviour?
Visual augmentation is about using AI to signal to humans when something out of the ordinary happens. BriefCam specialises in visually augmenting video data to allow humans to rapidly review video. Their Video Synopsis technology collects all objects from a target period to create a much shorter video, in which objects and activities that originally occurred at different times are displayed simultaneously – one hour of video can be reduced down to one minute. When you consider that the most filmed object in the world is a closed door, this type of time-saving technology is a true game changer in the world of surveillance.
Digital Barriers is another company working in this field and helping to optimise the use of body-worn cameras used by public safety and law enforcement professionals. By offering zero latency streaming and analysis of secure video and related intelligence over wireless networks, if the video content analysis system identifies the person confronting the officer as a known criminal, it can give an alert.
As an indicator of the way things are going, the Computer Science and Artificial Intelligence Laboratory at the Massachusetts Institute of Technology (MIT) has created a visual augmentation model that can predict human actions from what people are doing in the seconds before they do it. Researchers fed the program with 600 hours of YouTube videos to see if the model could learn about and predict certain human interactions like hugs, kisses, high-fives, and handshakes. Analysing a video of people who are seconds away from doing something like this, the computer managed a 43 per cent success rate, compared to 71 per cent reached by actual humans. MIT claims that it will be much more successful if it consumes more video data than the 600 hours used for the experiment.
Keeping pace with innovation
However, with technology advancing so fast it’s difficult for any single company to keep up and the future for video analytics companies is about combing skills, talent and vision.
By challenging the status quo and unleashing our imaginations, we are rapidly reaching a stage where machines are able to do what they’re best at, together with humans doing what we’re best at.
Combining our best features is important for our future. Why? The IoT allows us to join data from as many types of sensor as a single dataset. It’s also important because we combine the efficiency of machine intelligence with the credibility of human judgment and experience to turn this data into valuable information.
For this to take flight in the world of video content, innovators must work collaboratively to combine skills and share their knowledge with one another.
One way to do this, right now, is to join a community that champions innovation and big ideas and offers a collaborative support network to companies of all sizes. Working as a community of innovators, we will be able to achieve the perfect balance of machine intelligence and human judgement.
Neil Killick is regional leader for northern Europe at Milestone Systems. For more information, visit www.milestonesys.com
- National Cyber Force set to “transform” nation's cyber protection capabilities
- Taking Fire Safety to Another Level
- Third Party Vendor Risk Management: Why it Matters to Financial Institutions
- Digital engineering team wins top award for excellence in improving fire safety
- NFCC responds to Home Office fire statistics
- Landlord failed to take fire safety seriously
- Waking Watch Relief Fund re-opened for one month by central Government
- NFCC responds to second reading of Building Safety Bill in Parliament
- Directors at greater risk of identity fraud
- Joint US and UK statement on terrorism prevention
- Surveillance camera commissioner contract extended
- Dahua signs distribution deal with ADI
- From the editor
- Blog for FSM website
- Feeling Secure
- Smart home
- Playing by the rule - June 2017
- Feeling secure - June 2017
- Entries now open for the Safety and Health Excellence Awards
- Surveillance camera compliance passport launched